Driven by artificial intelligence and machine learning, the demand for edge computing is gradually increasing. Relevant institutions predict that the amount of data created, collected, and replicated globally will rapidly expand, reaching 175ZB (ZeByte) by 2025. To reduce the workload of data processing in the cloud, more and more data will also be placed on the edge side for computation.
As the main control chip of electronic devices, MCU plays an important role in improving the data processing and decision-making ability of edge computing devices. It will usher in new development opportunities and also face more challenges in the wave of edge AI.
01
Edge AI Wave Attacks, Expanding MCU Market Space
The demand in the fields of Internet of Things (IoT) devices, industrial control, smart homes, and smart wearables is driving the growth of the MCU market. In the long run, while ensuring low power consumption and strong real-time performance, it has become the expectation of downstream markets to enable MCU to have stronger edge side computing and intelligent decision-making capabilities.
In this process, edge AI has become a focus of intelligent device development. Edge AI is the deployment of AI applications on devices in the physical world. The reason why it is called "edge AI" is because this type of AI calculation is completed near the data location. Compared to cloud computing, edge AI has strong real-time capabilities, and data processing does not experience delays due to long-distance communication. Instead, it can respond more quickly to the needs of end users, while also ensuring data privacy and security.
Compared with the characteristic that traditional edge computing can only respond to pre completed program input, edge AI has more flexibility, which allows more diverse signal input (including text, voice and a variety of acousto-optic signals) and intelligent solutions for specific types of tasks.
The above characteristics of edge AI have a strong compatibility with MCU. On the one hand, MCU has the characteristics of low power consumption, low cost, real-time performance, and short development cycle, making it suitable for edge intelligent devices that are sensitive to cost and power consumption. On the other hand, the integration of artificial intelligence algorithms can also strengthen the MCU, enabling it to balance higher performance data processing tasks.
Therefore, "MCU+edge AI" is being applied in more and more fields such as image monitoring, speech recognition, and health monitoring. In addition, on the basis of IoT, the combination of MCU and edge AI will also promote the development of AIoT (Artificial Intelligence Internet of Things), enabling various devices to be more intelligent and interconnected. Data firm Mordor Intelligence predicts that the edge artificial intelligence hardware market will grow at a compound annual growth rate of 19.85% between 2024 and 2029, reaching $7.52 billion by 2029.
"The future development of MCUs will be oriented towards specialization and intelligence," Liu Shuai, Chief Marketing Officer of Beijing Yisiwei Computing Technology Co., Ltd., told China Electronics News. "Intelligence is reflected in two aspects: first, strengthening support for AI algorithms and machine learning models, enabling MCUs to have a certain level of intelligent decision-making ability; second, improving performance. High end products will adopt multi-core design to enhance processing capabilities and meet high-performance needs."
02
Integrated AI accelerator to enhance MCU performance
Edge AI has brought many market opportunities to MCU, and strengthening the AI performance of MCU is of utmost importance to meet the computing needs of intelligent devices for artificial intelligence on the edge side.
"Facing the needs of edge AI and end-to-end AI, MCU needs to make the following adjustments to enhance AI computing power." Chen Siwei, Product Marketing Director of the MCU Business Unit at Zhaoyi Innovation, stated, "Firstly, it integrates AI accelerators, such as neural network accelerators or specialized vector processors, to accelerate AI inference and training tasks; secondly, it optimizes energy efficiency ratio, reduces power consumption while maintaining performance, and extends device endurance; thirdly, it enhances security, including data encryption, secure boot, and secure storage, to protect user data from attacks; fourthly, it supports multimodal perception; fifthly, it optimizes system integration, providing more hardware interfaces and software support, making it easier for developers to integrate AI functions into edge devices."
In terms of AI accelerators, both digital signal processors (DSP) and neural network processors (NPU) have become important acceleration components integrated into the MCU, allowing the MCU to run AI algorithms at the edge. Specifically, DSP is more suitable for signal processing tasks, including audio, video, communication, etc., while NPU focuses more on efficiently processing a large number of matrix operations and parallel computing tasks.
For this reason, major manufacturers are actively laying out. STM32N6, launched by STM Semiconductor in 2023, adopts Arm Cortex-M55 core, integrates ISP and NPU to provide machine vision processing capability and AI algorithm deployment. NXP has launched the MCX N series MCU, which features a dual core Arm Cortex-M33 and integrates an eIQ Neutron NPU. It is understood that this NPU can improve machine learning inference performance by about 40 times.
As the supplier of most MCU cores, Arm also puts effort into the edge side NPUs. In April, Arm launched the Ethos U85 NPU as an AI micro accelerator that supports the Transformer architecture and CNN (Convolutional Neural Network). Combined with the Armv9 Cortex-A CPU, it can provide 4TOPS of end-to-end computing power to assist AI inference.
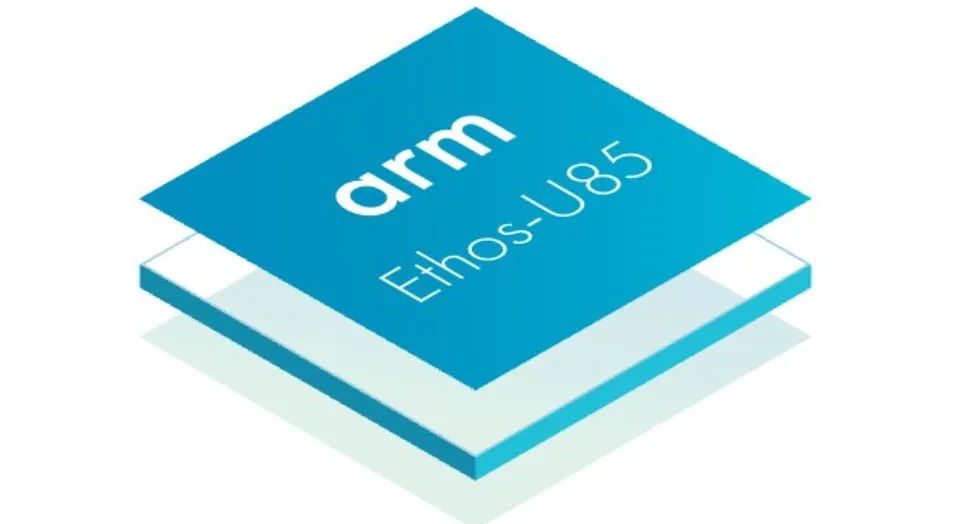
Arm Ethos U85 NPU (Image source: Arm)
"Many different processors can implement artificial intelligence. AI can run on Arm cores, NPUs, and DSPs, and different processors will run different algorithms, resulting in different power consumption." Rafael Sotomayor, Executive Vice President and General Manager of Security Connection Edge Business at NXP, said, "Whether to implement AI functionality in Arm cores or DSPs depends on the technical expertise of the engineering team. We use NPUs because they are faster (based on our technology) and require less energy. Of course, if customers have specific machine learning algorithms, they can continue to use DSPs."
03
Realize performance, power consumption, and cost balance,
Building a platform based solution
Although MCU can support AI algorithms by integrating AI accelerators such as NPUs, high performance often leads to higher power consumption. Therefore, while improving performance, it is also necessary to ensure that the MCU achieves a balance between many factors such as power consumption and cost. This not only tests the chip design capabilities of MCU manufacturers, but also puts higher demands on the overall cost and power optimization of the company.
Using self-developed NPUs has become one of the choices for manufacturers to balance cost and efficiency. It is reported that STM32N6 released by STF Semiconductor adopts self-developed Neural Art accelerator, and the eIQ Neutron NPU integrated in NXP MCX N series is also self-developed. This is not only beneficial for reducing authorization costs, but also ensures reasonable control of the NPU iteration rhythm within its own technical path.
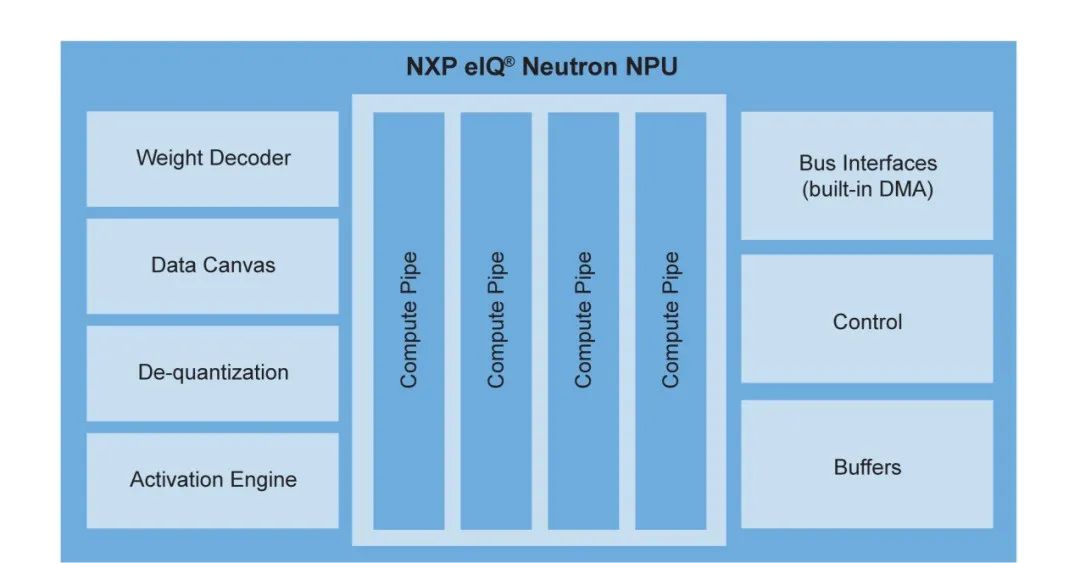
Block diagram of eIQ Neutron neural processing unit (Image source: NXP)
Meanwhile, the options for kernel architecture are also increasing. Currently, in the MCU market, except for a small number of 8-bit MCUs that use CISC architecture, the Cortex-M series cores with Arm architecture occupy the mainstream due to their good power consumption performance. With the gradual development of RISC-V architecture, this emerging architecture has also gradually gained the favor of manufacturers. It is reported that the MAX78000/2 launched by ADI provides two solutions, Arm core and RISC-V core, on the basis of integrating a dedicated neural network accelerator. It can perform AI processing locally at low power consumption, minimizing the energy consumption and delay of CNN operations.
Liu Shuai told reporters that adopting the RISC-V architecture can bring more advantages to MCU manufacturers. On the one hand, the RISC-V core is flexible and customizable according to specific application needs; On the other hand, due to the simpler RISC-V architecture and lower power consumption. In addition, compared to the ARM architecture, RISC-V, as an open-source architecture, can also reduce licensing and development costs.
From the customer's perspective, in addition to achieving a balance between high performance, low power consumption, low cost, and other aspects of MCU products, we also hope that MCU manufacturers can provide a complete platform based solution.
"In the past, customers' choices were bottom-up - they first chose chips and then thought about what software, applications, etc. they needed. Now, they start from the application layer and go down to the chips to obtain technical support." Rafael Sotomayor pointed out that "these technologies are very complex, involving not only artificial intelligence, but also information security, functional security, vision, audio, etc.".
Therefore, helping customers simplify the complexity of technology has become the core value for manufacturers to provide products and services to customers In April of this year, NXP announced a partnership with Nvidia to integrate Nvidia's TAO working components into the NXP eIQ machine learning development environment for developers to accelerate development and deploy trained AI models.
ADI provides the development tool MAX78000 EVKIT # based on MAX78000/2 to help developers achieve a smooth evaluation and development experience. STM32Cube, a cloud developer platform, has also been launched by STF Semiconductor AI, Support users to configure existing resources in the cloud, further reducing the complexity of edge artificial intelligence technology development.
Overall, facing the challenges brought by the edge AI wave, MCU manufacturers are actively exploring and demonstrating diverse development paths. Although the "optimal solution" of architecture, AI accelerator and other solutions has not been determined yet, it is the trend for MCU to continuously improve its computing capability and security performance to adapt to the increasingly complex edge computing environment on the premise of ensuring the basic characteristics of low power consumption and low cost.