When it comes to artificial intelligence systems, we rarely see such things: the price tag of accelerators and the basic motherboard that glues a bunch of accelerators together to form a shared computing complex.
But at the recent Computex IT conference held in Taipei, Taiwan, Intel, eager to showcase its expertise in AI training and reasoning, did something that Nvidia and AMD had not done before: providing pricing for its current and previous generations of AI accelerators. We expect Nvidia, AMD, or any other AI accelerator and system startups not to follow suit soon, so don't get too excited.
However, the pricing of Gaudi 2 and Gaudi 3 accelerators, as well as some benchmark test results and disclosure of peak feed and speed of these machines, provide us with the opportunity to conduct some competitive analysis.
The reason why Intel discusses its pricing is simple. The company is attempting to sell some artificial intelligence chips to offset the cost of its future "Falcon Shores" GPU being put into use by the end of 2025 and the subsequent "Falcon Shores 2" GPU being launched in 2026. Therefore, it must demonstrate good cost-effectiveness and competitive performance.
This is particularly important because the Gaudi 3 chip began shipping in April and is the terminator of the Gaudi accelerator series acquired by Intel after acquiring Habana Labs for $2 billion in December 2019.
Due to the extremely high heat generation and manufacturing costs of the Ponte Vecchio Max series GPUs (which are the core of the Aurora supercomputer at the Argonne National Laboratory and have been installed on several other machines and almost immediately sealed after completing these transactions), Intel is attempting to bridge the gap between the long delayed Ponte Vecchio and the expected timely launch of Falcon Shores by the end of next year.
As revealed by Intel in June 2023, the Falcon Shores chip will adopt the Gaudi series of large-scale parallel Ethernet architecture and matrix mathematical units, and merge it with the Xe GPU engine created for Ponte Vecchio. In this way, Falcon Shores can perform both 64 bit floating-point processing and matrix mathematical processing simultaneously. Ponte Vecchio does not have matrix processing, only vector processing, which was intentionally done to meet Argonne's FP64 requirements. This is great, but it means that Ponte Vecchio may not be suitable for AI workloads, which limits its appeal. Therefore, Gaudi and X e computing units are merged into a single Falcon Shores engine.
We don't know much about Falcon Shores, but we know its weight is 1500 watts, which is 25% higher than the power consumption and heat dissipation of the top tier "Blackwell" B200 GPU expected to be shipped in bulk early next year. The latter has a rated power of 1200 watts and provides 20 petaflops of computing power with FP4 accuracy. When consuming 25% more power, Falcon Shores performs at least 25% better than Blackwell at the same floating-point accuracy level and roughly the same chip manufacturing process level. Even better, Intel would prefer to use its Intel 18A manufacturing process, which is expected to be put into production in 2025, to manufacture Falcon Shores, and its floating-point performance would be even stronger than this. The Falcon Shores 2 is best equipped with a smaller Intel 14A process and is expected to be put into production in 2026.
Intel should have stopped wasting time on OEM and chip design businesses long ago. TSMC's innovation pace is ruthless, and Nvidia's GPU roadmap is also unwavering. The "Blackwell Ultra" in 2025 will bring an upgrade to HBM memory and may also enhance GPU computing power. The "Rubin" GPU will be launched in 2026, and subsequent products of the "Rubin Ultra" will be launched in 2027.
Meanwhile, Intel announced in October last year that its Gaudi accelerator sales channel was worth $2 billion, and added in April this year that it expects Gaudi accelerator sales to reach $500 million in 2024. This is negligible compared to AMD's projected $4 billion in GPU sales this year (which we believe is too low, more likely to be $5 billion) or Nvidia's potential $100 billion or more in revenue in data center computing this year (only data center GPUs, no networks, no DPUs). But clearing the $2 billion channel means paying for Falcon Shores and Falcon Shores 2, so Intel's enthusiasm is high.
Therefore, Intel announced pricing and developed benchmark tests at its Computex briefing to demonstrate the competitiveness of the Gaudi 3 compared to the current "Hopper" H100 GPU.
Intel's first comparison was focused on AI training, targeting the GPT-3 large language model with 175 billion parameters and the Llama 2 model with 70 billion parameters:
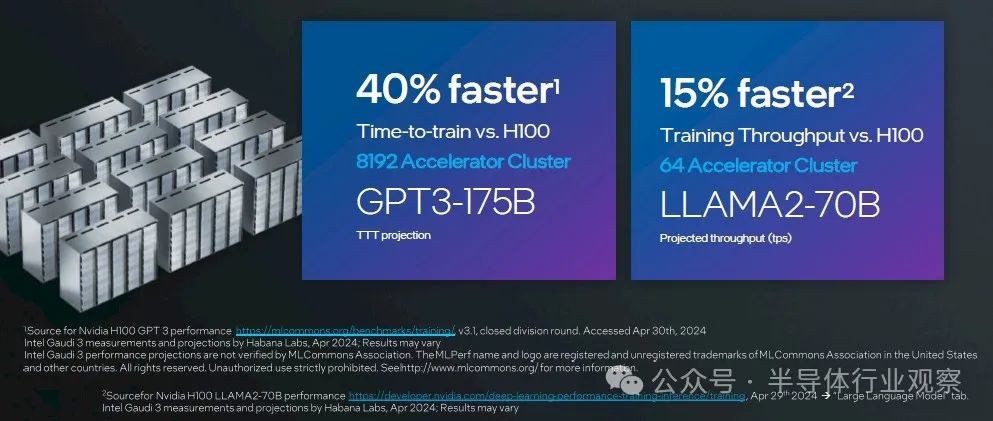
The above GPT-3 data is based on MLPerf benchmark testing, while the Llama 2 data is based on Nvidia's H100 results and Intel's estimates. The GPT benchmark runs on a cluster with 8192 accelerators - Intel Gaudi 3 has 128 GB HBM, while Nvidia H100 has 80 GB HBM. The Llama 2 test runs on a machine with only 64 devices.
For the sake of reasoning, Intel conducted two comparisons: one comparing the Gaudi 3 with 128 GB HBM to the H100 with 80 GB HBM in a series of tests, and the other comparing the Gaudi 3 with the same 128 GB of memory to the H200 with 141 GB HBM. Nvidia data is published here for various models using TensorRT inference layers. Intel data is predicted for Gaudi 3.
Here is the first comparison between H100 80 GB and Gaudi 3 128 GB:
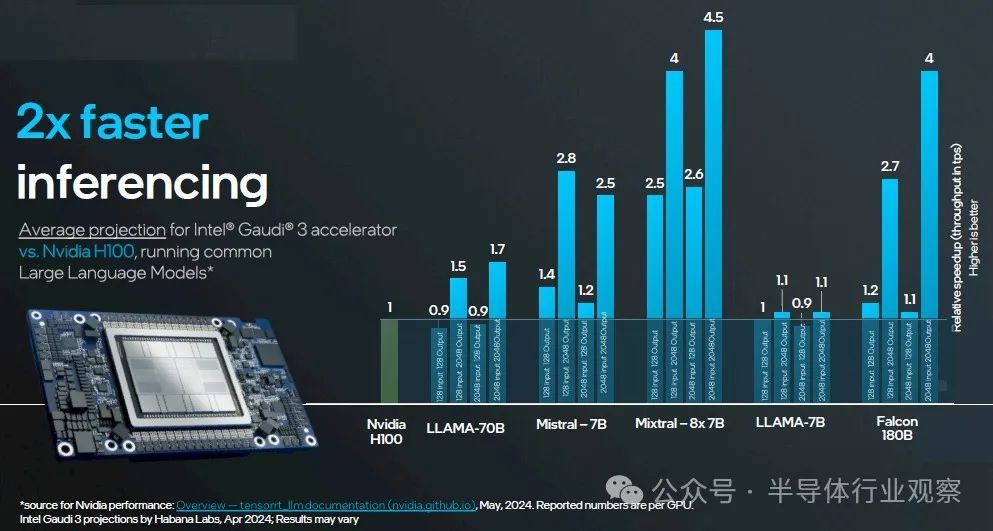
Here is the second comparison, H200 141 GB and Gaudi 3 128 GB:
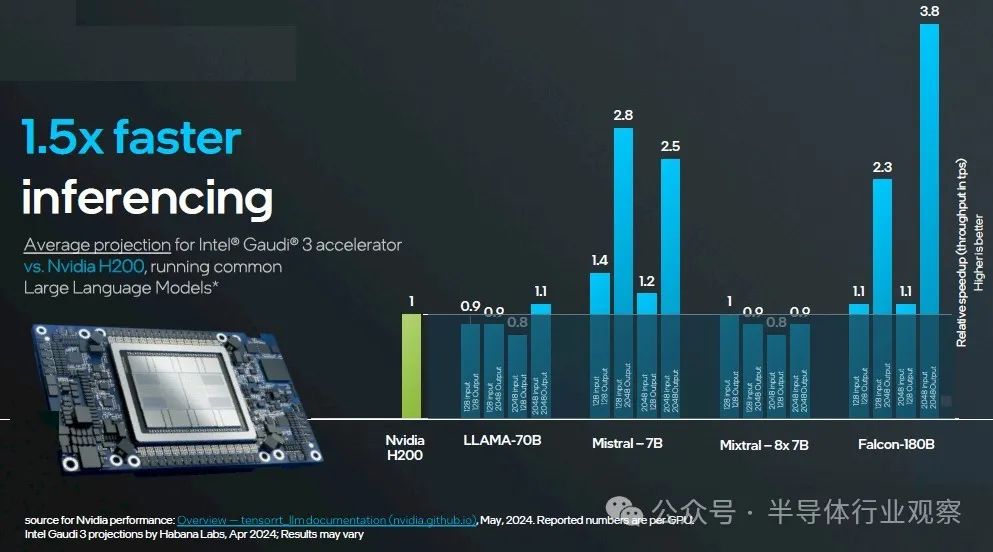
We will remind you of two things we have mentioned throughout the entire AI craze. Firstly, providing the best value for money AI accelerator is truly what you can get. Secondly, if it can perform matrix mathematical operations with reasonable precision combinations and run the PyTorch framework and Llama 2 or Llama 3 models, then you can sell it because Nvidia GPU supply is insufficient.
But for Intel, this is an opportunity to make money:
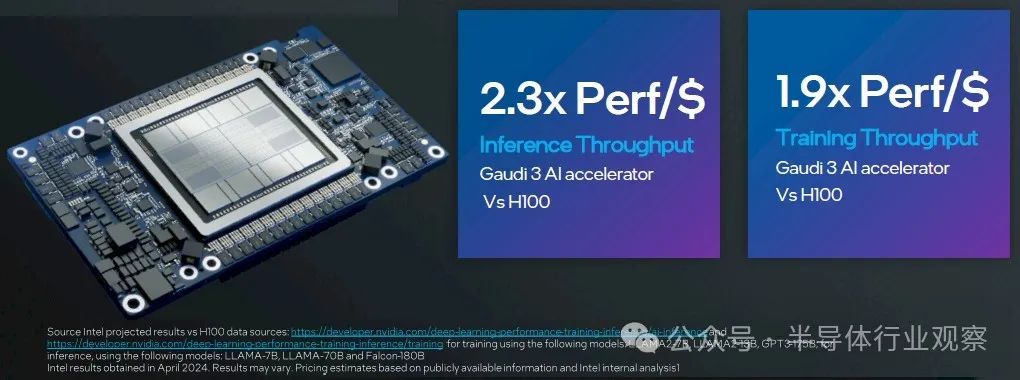
During the training process, Intel compared the average of Nvidia's real data (Llama 2 7B, Llama 2 13B, and GPT-3 175B tests) with Intel's estimate of Gaudi 3. During the inference process, Intel used the average of Nvidia's real data (Llama 2 7B, Llama 2 70B, and Falcon 180B) and Gaudi 3's estimated values.
If you perform reverse calculations on these performance/dollar ratios and the relative performance data displayed in the chart, Intel assumes the cost of the Nvidia H100 accelerator to be $23500, while if we perform simple calculations on the Gaudi 3 UBB, the cost would be $15625.
We like to observe trends over a period of time and broader peak theoretical performance in order to find out who has higher cost-effectiveness. Therefore, we created a small table comparing Nvidia "Ampere" A100, H100, and Blackwell B100 with Intel Gaudi 2 and Gaudi 3 accelerators, both of which use a substrate configuration with eight accelerators. Please take a look at this:
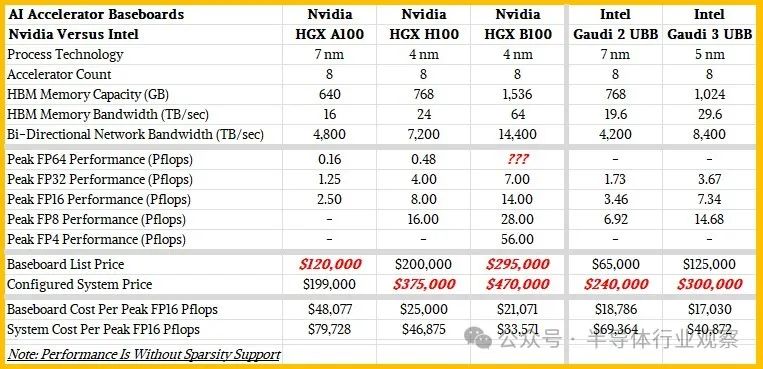
Please remember, these are the numbers of the eight way motherboard, not the numbers of the device, which will become the basic computing unit for most AI customers today.
Of course, we are fully aware that each AI model has its own uniqueness in utilizing the computing, memory, and network of these devices and their substrate clusters. The mileage will definitely vary depending on workload and settings.
We also like to think from a system perspective. We have estimated the cost of using these substrates and adding a dual slot X86 server complex, which has 2 TB of main memory, 400 Gb/s InfiniBand network card, a pair of 1.9 TB NVM Express flash drives for the operating system, and eight 3.84 TB NVM Express flash drives for storing local data to UBB.
Our table shows the relative cost-effectiveness of these five machines. We use FP16 accuracy to measure all of these devices, which we believe is a good benchmark for comparison, and there is no sparsity support activated on the devices because not all matrices and algorithms can take advantage of this. If you want to do mathematical calculations yourself, you can use lower accuracy.
According to Huang Renxun's keynote speech last year, the cost of HGX H100 substrate is $200000, so we actually know this number, which is consistent with the complete system pricing we see in the market. Intel just informed us that the cost of a substrate with eight Gaudi 3 accelerators is $125000. The rated speed of the H100 substrate is 800 million floating-point operations, while the rated speed of the Gaudi 3 substrate is 734 million floating-point operations, with FP16 accuracy and no sparsity. This means that the cost of every 10 billion floating-point operations for the H100 complex is $25000, while the cost of every 10 billion floating-point operations for the Gaudi 3 is $17030, which is 32% more cost-effective and beneficial to Intel.
Now, if you build a system and add those expensive CPUs, main memory, network interface cards, and local storage, the gap will start to narrow. According to the configuration we outlined above, the cost of the Nvidia H100 system may be approximately $375000, which is $46875 per billion floating-point operations. The cost of a Gaudi 3 system with the same configuration is approximately $300000, with a cost of $40872 per billion floating-point operations. This is only 12.8% more cost-effective than the Nvidia system.
If the same exchange, support, electricity, environment, and management costs are added, the gap will become smaller.
Therefore, please think at the system level and benchmark your own models and applications.
Now, one last thing: let's talk about the revenue and channels of Intel Gaudi 3. If you calculate, $500 million is just 4000 substrates and 32000 Gaudi 3 accelerators. And the remaining $1.5 billion in the Gaudi channel is almost entirely used for potential sales of Gaudi 3 devices - rather than unfinished sales backlog, so it is definitely not a cat in the bag - and only represents an opportunity to sell 12000 substrates and a total of 96000 accelerators. Nvidia will sell millions of data center GPUs this year, although many of them will not be H100, H200, B100, and B200, many of them will be.